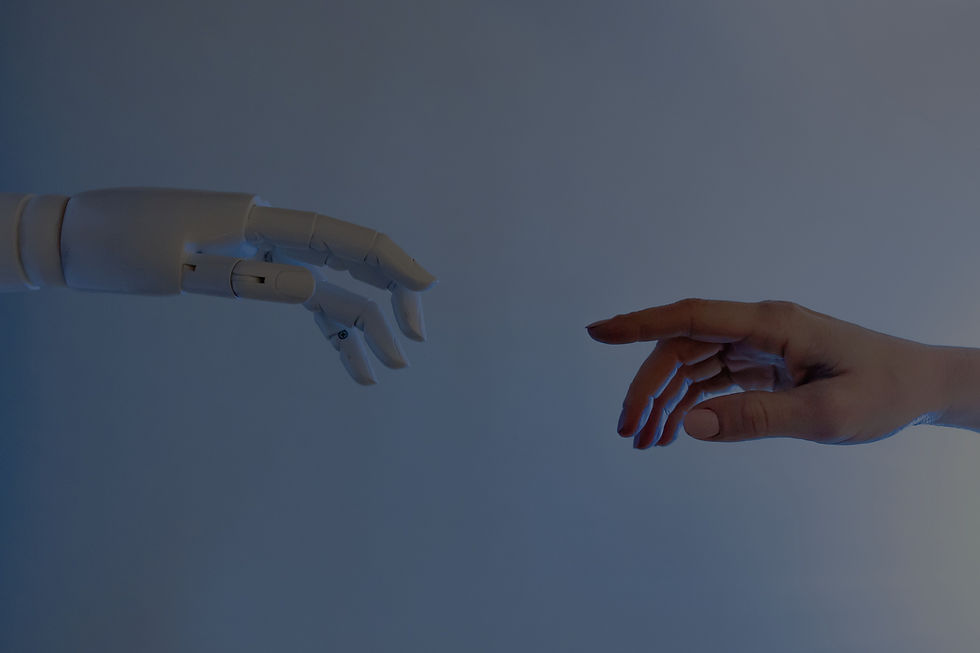
Overview
This digital journal examines three learning innovations, and forms part of an assignment for my studies in Future Trends of Learning Design at QUT.
1. Introduction
In this assignment I critically evaluate three learning innovations, then reflect on their value in my own work and for the field of learning design in the future, with reference to insightful peer contributions in discussion forums.
ElevenLabs' Prime Voice AI combines TTS with AI to create remarkably expressive human-like narration.
Another feature allows users to clone their own voice for narration.
​
(Note: video has sound. Screen recording taken of author trialling Prime Voice AI, April 6, 2023 at https://beta.elevenlabs.io/speech-synthesis)
2.1 Text-to-speech technology
With around 16% of the world’s population experiencing disability today - a rate that is rising (WHO, 2021) - there is a growing need to help people with disabilities access education (Abdi et al., 2021). Text-to-speech (TTS) tools are one type of assistive technology (AT) that can address this need by helping people compensate for reading difficulties (Wood et al., 2018). By converting written text to spoken text, TTS tools improve access for people with disabilities including visual impairments, dyslexia, aphasia and other learning disabilities (Young et al., 2019). Widely available as web browser extensions, mobile apps, software and built into operating systems, TTS has a shown a number of benefits and limitations in learning contexts.
Among its advantages, increasing educational access using TTS tools has been shown to improve reading rates, reading comprehension, learning outcomes and engagement among students with a range of learning and reading difficulties (Gruner et al., 2017; Young et al., 2019). Such positive outcomes seem easily supported in digital and online learning, as TTS tools provide a readily applicable method to align with Universal Design for Learning (UDL) principles (Rao, 2021). For example, online courses can integrate the use of TTS browser extensions, which provide “a powerful way to support students with reading skills such as decoding, fluency, and comprehension” (Rao, 2021, para. 28).
Besides advantages, TTS does have limitations. One of the biggest is the tendency to output a monotonous, even tonally negative synthetic voice, which can be demotivating to users (Hillaire et al., 2019). This is a key problem for online learning; in fact, one of Mayer’s well-supported principles of multimedia, the voice principle, posits that deeper learning occurs when voice narration is human rather than machine-created (Mayer, 2014). Interestingly, research has also shown that more unnatural-sounding TTS voices not only impose a higher cognitive load on listeners, but that listeners respond most positively to TTS voices that represent personality traits similar to their own, for example extroversion or introversion (Cambre et al., 2020, p.2). Nonetheless, some cutting-edge TTS technology appears to match real human voices in expression of emotion, tone and resulting learning outcomes (Craig & Schroeder, 2019). In particular, recent advances in artificial intelligence (AI) human voice cloning can be combined with TTS technology to produce remarkably human-like TTS narration, which suggests tantalising future applications of TTS in learning design, such as removing the need to record voiceover for multimedia, and availing of voice cloning AI to allow learners to listen to texts read out not just by a TTS voice with similar personality traits, but in the learner’s own voice.
Game elements (incl. scores and feedback) in 'Snakebite Hero' - a learning experience I designed for snakebite first aid training, and refer to in the reflection section of this digital journal. Click here to try it yourself. (Video above has sound).
2.2 Gamification
​
Gamification refers to the use of aspects of games such as scores, badges, challenges and leaderboards in non-game contexts (Jang et al., 2015). Since 2010, the perceived potential of gamification to enhance online learning experiences has generated growing excitement and research interest (Hamari et al., 2014). However, studies have shown mixed results, with positive outcomes often appearing to depend heavily on students’ individual differences and the learning context (Antonaci et al., 2019). With its emphasis on external rewards, gamification might at first seem a notably behaviourist approach to reinforcing desired behaviours, however its encouragement of mastery, together with the use of badges which can encourage a sense of community among badge earners, also align with social-cognitive perspectives of learner motivation (Sailer et al., 2013).
Among its benefits in online learning contexts, gamification can result in greater learner motivation and engagement (Atonaci et al., 2019; Park & Kim, 2021). Innovative applications include awarding points and badges for activity completion and learning goal achievement, while leaderboards can be used to encourage positive social pressure among learners (Bovermann & Bastiaens, 2020). This concept of social pressure is central to many aspects of gamification; by encouraging social comparison, the aim is to spark learners' competitive drive to perform vs. other learners, which has been shown to support learner motivation and engagement (Antonaci et al., 2019).
There are pitfalls related to gamification, however. Firstly, in the context of adult online learning the use of the external rewards of game mechanics appears in some cases to be demotivating for more intrinsically motivated learners (Leung et al., 2022). This makes sense through the lens of the self-determination theory of motivation, which posits that while external rewards can be powerful motivators for students who have a less internalised drive to learn, they can in fact detract from more internally-driven students’ motivation, by shifting the focus from internal to external reinforcements (Ryan & Deci, 2017).
A further issue is the potential for gamification to pose barriers to learning for people with disabilities (Smith & Abrams, 2019). Though this problem appears to be drastically under-researched in the literature, it seems clear that gamification’s emphasis on performance and social comparison could alienate learners whose disabilities might hinder them from achieving scores and appearing high on student leaderboards. This further highlights the need for learning designers to avoid incorporating gamification uncritically; we must be wary of alienating some learners for the sake of engaging and motivating others. To that end, decisions to include game elements - as with all learning design choices - should be made with UDL principles in mind (Cumming & Rose, 2022).
Using ChatGPT as a virtual writing assistant.
(Screen recording taken by the author featuring OpenAI's ChatGPT https://openai.com/blog/chatgpt.
Copied text is a real example of writing by a student with lower-intermediate level English writing skills, and was extracted from the Victorian Department of Education's website: https://www.education.vic.gov.au/school/teachers/support/diversity/eal/companion/Pages/B1-Writing.aspx)
2.3 AI - Large Language Models
Large Language Models (LLMs) such as ChatGPT and GPT-4 are powerful new forms of generative artificial intelligence (AI) that are trained to produce human-like language in response to instructions (OpenAI, 2023). They achieve this by using machine learning algorithms to harvest information and language patterns from vast amounts of digital text, including from websites, news media, books and Wikipedia (Cooper, 2023, p.2). In the short time since the release of ChatGPT and similar LLMs, they have captured the public’s attention and have come to be viewed by many as potentially transformative in various areas of society, not least in the field of education (Cooper, 2023). Public and academic discourse on their uses in education abounds, which has served to highlight potential benefits and pitfalls.
Among their advantages, LLMs may represent innovative tools to improve learning outcomes. For example, an LLM could improve student performance by acting as a virtual writing assistant, providing “automated and responsive feedback to students as they study and complete course assignments and tasks” (Pelletier et al., 2022, p.20). They could also help university students with efficient research, by producing summaries and highlighting key aspects of research literature that can help them gain a quick understanding (Kasneci et al., 2023). Using LLMs in this way could be seen as an innovative social constructivist practice, in that the student can be guided towards mastery through what Vygotsky (1978) termed the ‘Zone of Proximal Development’ by an AI guide, or – if the LLM harvests data from user inputs – the student might even be seen as co-constructing knowledge with an AI ‘peer’.
LLMs could also be used as assistive technology to improve access and equity in education. For example, students with physical, cognitive or literacy challenges could benefit greatly from feedback, guidance and correction of their writing provided by an LLM (Kasneci et al., 2023). Meanwhile, Kuzdeouv et al. (2023) demonstrate how combining ChatGPT with speech-to-text and text-to-speech technology removes barriers for the visually impaired to access ChatGPT’s benefits.
Learning designers and educators can also benefit from LLMs. For example, generative AI can reduce workload by drafting course outlines, content, learning outcomes and assessments for online learning design (Gilmore et al., 2023). Similarly, teachers can use LLMs to prepare lesson plans, step in to provide student writing feedback, and have learners debate with chatbots like ChatGPT to improve their debating skills (Roose, 2023).
Despite the positives, many are concerned about potential downsides. One major issue is the threat to academic integrity, as students can simply plagiarise entire essays with reduced fear of detection (Khalil & Er, 2023). This represents a serious challenge in education, given that written work is widely used to assess learners. However, some argue that rather than despair, we can view LLMs as not only an inevitable part of future educational practice, but also as a catalyst for positive change; we can take it as an opportunity to innovate approaches to assessment, moving away from what might be seen as the outdated reliance – particularly in higher education - on essays (Rudolph et al., 2023).
Further problems with LLMs arise in their tendency to ‘hallucinate’ (make seemingly realistic yet completely false assertions) – and perpetuate discriminatory sexual, religious and racial biases due to their echoing of human biases present in online text (Cooper, 2023, p.2). These issues point to the need to exercise caution in practice, as well as the need to train all stakeholders in appropriate and ethical use of generative AI in educational contexts (Rudolph et al., 2023).
3. Reflection
In this section I briefly reflect on each learning innovation and engage with my peers’ thoughts from discussion boards.
3.1 Text-to-speech technology
As Marlee correctly noted in the discussion board, designing for disability first tends to benefit all learners (M. Follman, personal communication, March 14, 2023), and on reflection my learning designs have been made more accessible by later modifications rather than initial planning. Examining TTS tools together with peers clarified for me the need to make design choices that support screen readers and TTS technology a standard feature of my work. To do this, I will use simple strategies such as providing alt text for non-text content, ensuring text content doesn’t rely on images for comprehension, and supporting keyboard compatibility, in alignment with WCAG 2.1 guidelines (W3C WAI, 2019).
Finally, Anne’s reply to my post was thought provoking; she highlighted that TTS appears less effective in supporting students’ inferential reading comprehension, which may still require teacher/facilitator guidance (A. Carran, personal communication, March 19, 2023). I agree, however, I believe that advances in TTS voice narration that allow for more human-like intonation and emphasis might better help learners – with or without disabilities – better understand inference in texts, which could offer opportunities to incorporate advanced TTS tools to support all students’ reading comprehension in my future learning design work.
3.2 Gamification
Based on user feedback, users have loved the game elements I have incorporated in my learning designs, for example in a ‘serious game’ eLearning I created on snakebite first aid with interactive video, scoring and feedback. However, reflecting now I again see that I didn’t adequately consider accessibility. To align with UDL principles and WCAG 2.1 standards, I could have designed in more flexibility, so that the module was engaging for learners whether completed with the video and audio elements or only using text; applying the abovementioned strategies to cater for TTS tools and providing audio narration of text content would also have been helpful. Adding to this, aside from the ubiquity of mobiles in any case, I agree with Richard’s comment in the forums that mobile-friendly design can improve equitable access, as low-income learners may not have access to desktop computers (R. Scharges, personal communication, March 28, 2023). This is another aspect I will better consider in my design approach in the future
Bridgitte’s question in the discussion forum raises another key issue: how can we assess real learning when using gamification? (B. Norris, personal communication, March 17, 2023). This speaks to the danger of favouring ‘fun’ elements without considering meaningful learning outcomes. My response to this is that we can include assessments that test learners’ ability to apply the knowledge in higher-order thinking tasks (as per Bloom’s Taxonomy), such as analysing, evaluating and creating solutions to real world problems.
3.3 Large Language Models
In my time teaching in higher education, I dealt with a lot of plagiarism in students’ written work, which lead me to view new generative AI chatbots such as ChatGPT with alarm for their cheating potential. However, researching the literature and engaging with my peer’s thoughts for this assignment has resulted in a minor revolution in my thinking on the matter. As Anne notes, though plagiarism is clearly a concern, generative AI has enormous potential as an assistive technology (A. Carran, personal communication, April 4, 2023), while Tina correctly pointed out its potential to improve workflow for learning designers and educators (T. Eisenmenger, personal communication, March 26, 2023), and Sally (S. Burchard, personal communication, March 18, 2023) aptly highlighted its benefits for language learners, all of which echoes academic commentary on the issue.
As expressed in my critical evaluation, though caution is appropriate, generative AI can also provoke a positive shift from a focus on written work towards more innovative, and perhaps more meaningful and inclusive assessment approaches. This new mindset and consideration of the positive opportunities presented by generative AI will inform my future learning design choices, and will, I believe, be a fruitful avenue to explore innovative learning approaches with clients.
​
Note: Reference list included in assignment submission.